The Impact of Technology on Financial Planning and Analysis
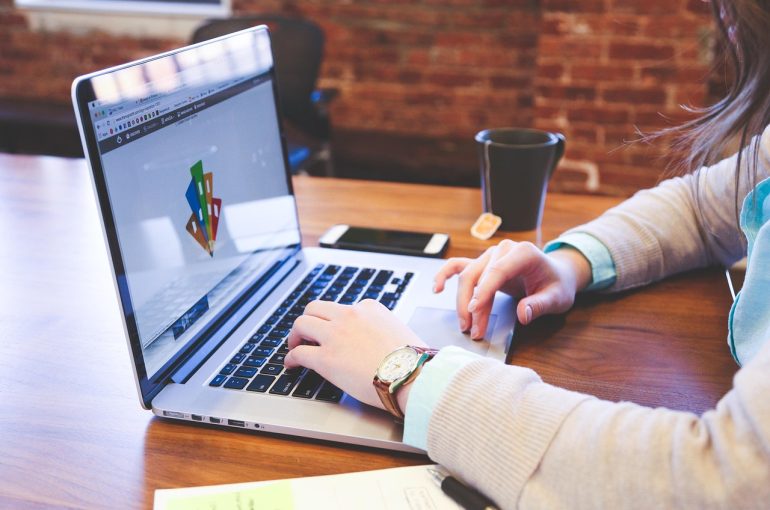
The Impact of Technology on Financial Planning and Analysis
Introduction to AI and Machine Learning in Financial Planning
Understanding AI and Machine Learning
Artificial Intelligence (AI) and Machine Learning (ML) are transformative technologies that are reshaping various industries, including financial planning and analysis. AI refers to the simulation of human intelligence processes by machines, especially computer systems. These processes include learning, reasoning, and self-correction. Machine Learning, a subset of AI, involves the use of algorithms and statistical models that enable computers to perform tasks without explicit instructions, relying on patterns and inference instead.
The Role of AI and ML in Financial Planning
AI and ML are increasingly being integrated into financial planning to enhance decision-making processes, improve efficiency, and provide deeper insights. These technologies can process vast amounts of data at unprecedented speeds, allowing financial analysts to identify trends, forecast future scenarios, and make data-driven decisions. By automating routine tasks, AI and ML free up human resources to focus on more strategic activities.
Key Applications in Financial Planning
Predictive Analytics
Predictive analytics is one of the most significant applications of AI and ML in financial planning. By analyzing historical data, these technologies can predict future financial trends, helping organizations to anticipate market changes and adjust their strategies accordingly. This capability is crucial for risk management and strategic planning.
Automated Reporting
AI and ML can automate the generation of financial reports, reducing the time and effort required to compile and analyze data. Automated reporting tools can provide real-time insights and visualizations, enabling financial planners to quickly assess the financial health of an organization and make informed decisions.
Risk Management
AI and ML are instrumental in enhancing risk management practices. By analyzing large datasets, these technologies can identify potential risks and anomalies that may not be apparent through traditional analysis methods. This proactive approach allows organizations to mitigate risks before they escalate into significant issues.
Benefits of AI and ML in Financial Planning
Enhanced Accuracy and Efficiency
AI and ML improve the accuracy of financial forecasts and analyses by minimizing human error and bias. The automation of routine tasks also leads to increased efficiency, allowing financial planners to focus on strategic decision-making.
Real-Time Insights
The ability of AI and ML to process and analyze data in real-time provides financial planners with up-to-date insights, enabling them to respond swiftly to changing market conditions and make timely decisions.
Cost Reduction
By automating labor-intensive tasks, AI and ML can significantly reduce operational costs. This cost efficiency is particularly beneficial for organizations looking to optimize their financial planning processes without compromising on quality or accuracy.
Challenges and Considerations
Data Quality and Security
The effectiveness of AI and ML in financial planning heavily depends on the quality and security of the data being used. Ensuring data accuracy, completeness, and protection against breaches is crucial for reliable outcomes.
Integration with Existing Systems
Integrating AI and ML technologies with existing financial systems can be challenging. Organizations must ensure compatibility and seamless integration to fully leverage the benefits of these technologies.
Skill and Expertise
The successful implementation of AI and ML in financial planning requires skilled professionals who understand both the technological and financial aspects. Investing in training and development is essential to build a workforce capable of harnessing these advanced technologies.
The Evolution of Financial Planning and Analysis
Early Stages of Financial Planning and Analysis
Manual Processes and Basic Tools
In the early stages of financial planning and analysis (FP&A), organizations relied heavily on manual processes and basic tools. Financial data was often recorded and analyzed using paper-based systems, which were time-consuming and prone to errors. The primary tools available were calculators and rudimentary spreadsheets, which limited the scope and accuracy of financial analysis.
Role of Accountants and Financial Analysts
During this period, the role of accountants and financial analysts was primarily focused on bookkeeping and basic financial reporting. Their responsibilities included maintaining accurate financial records, preparing financial statements, and ensuring compliance with regulatory requirements. The emphasis was on historical data analysis, with limited forward-looking insights.
Introduction of Computerized Systems
Emergence of Spreadsheets
The introduction of computerized systems marked a significant shift in FP&A. The emergence of spreadsheet software, such as VisiCalc and later Microsoft Excel, revolutionized the way financial data was managed and analyzed. These tools enabled more efficient data entry, calculation, and analysis, allowing financial professionals to perform complex financial modeling and scenario analysis.
Automation of Routine Tasks
With the advent of computerized systems, routine tasks such as data entry, reconciliation, and report generation became automated. This automation reduced the time and effort required for manual processes, allowing financial analysts to focus on more strategic activities. The increased efficiency also improved the accuracy and reliability of financial data.
Integration of Enterprise Resource Planning (ERP) Systems
Centralization of Financial Data
The integration of Enterprise Resource Planning (ERP) systems further transformed FP&A by centralizing financial data across the organization. ERP systems provided a unified platform for managing financial transactions, budgeting, and reporting. This centralization improved data consistency and accessibility, enabling more comprehensive and accurate financial analysis.
Enhanced Reporting and Analysis Capabilities
ERP systems enhanced reporting and analysis capabilities by providing real-time access to financial data. Financial analysts could generate detailed reports and dashboards, facilitating better decision-making and strategic planning. The ability to drill down into specific data points allowed for more granular analysis and insights.
Advent of Business Intelligence and Analytics
Data-Driven Decision Making
The advent of business intelligence (BI) and analytics tools marked a new era in FP&A. These tools enabled organizations to harness the power of data for decision-making. Financial analysts could leverage advanced analytics techniques, such as data mining and predictive modeling, to uncover trends, identify opportunities, and mitigate risks.
Visualization and Dashboarding
BI tools introduced visualization and dashboarding capabilities, allowing financial professionals to present complex data in a more intuitive and accessible format. Interactive dashboards provided real-time insights into key performance indicators (KPIs) and financial metrics, facilitating more informed and agile decision-making.
Emergence of Artificial Intelligence and Machine Learning
Predictive and Prescriptive Analytics
The emergence of artificial intelligence (AI) and machine learning (ML) has further evolved FP&A by enabling predictive and prescriptive analytics. AI and ML algorithms can analyze vast amounts of data to identify patterns and predict future outcomes. This capability allows financial analysts to develop more accurate forecasts and optimize financial strategies.
Automation of Complex Analysis
AI and ML have also automated complex financial analysis tasks, such as anomaly detection, risk assessment, and portfolio optimization. By automating these tasks, financial professionals can focus on strategic planning and value-added activities. The integration of AI and ML into FP&A processes has improved efficiency, accuracy, and agility in financial decision-making.
Key Benefits of AI and Machine Learning in Finance
Enhanced Data Analysis and Insights
AI and machine learning technologies enable financial institutions to process vast amounts of data quickly and accurately. These technologies can identify patterns and trends that are not immediately apparent to human analysts. By leveraging machine learning algorithms, financial analysts can gain deeper insights into market behaviors, customer preferences, and investment opportunities. This enhanced data analysis capability allows for more informed decision-making and strategic planning.
Improved Risk Management
AI and machine learning play a crucial role in enhancing risk management practices within the financial sector. These technologies can analyze historical data and current market conditions to predict potential risks and assess their impact. Machine learning models can continuously learn and adapt to new data, improving their accuracy over time. This allows financial institutions to proactively manage risks, such as credit risk, market risk, and operational risk, thereby safeguarding their assets and ensuring regulatory compliance.
Automation of Routine Tasks
The automation of routine and repetitive tasks is one of the most significant benefits of AI and machine learning in finance. By automating processes such as data entry, transaction processing, and report generation, financial institutions can reduce operational costs and minimize human error. This automation frees up valuable time for financial professionals, allowing them to focus on more strategic and value-added activities, such as financial planning and analysis.
Personalized Customer Experiences
AI and machine learning enable financial institutions to offer personalized customer experiences by analyzing customer data and behavior. These technologies can tailor financial products and services to meet individual customer needs and preferences. For example, AI-driven chatbots can provide personalized financial advice and support, enhancing customer satisfaction and loyalty. Personalized experiences not only improve customer engagement but also drive revenue growth for financial institutions.
Fraud Detection and Prevention
AI and machine learning are instrumental in detecting and preventing fraudulent activities in the financial sector. These technologies can analyze transaction patterns and identify anomalies that may indicate fraudulent behavior. Machine learning models can continuously learn from new data, improving their ability to detect emerging fraud patterns. By implementing AI-driven fraud detection systems, financial institutions can protect themselves and their customers from financial losses and reputational damage.
Enhanced Forecasting and Predictive Analytics
AI and machine learning enhance forecasting and predictive analytics capabilities in finance. These technologies can analyze historical data and current market trends to generate accurate forecasts and predictions. Financial institutions can use these insights to anticipate market movements, optimize investment strategies, and make informed decisions. Enhanced forecasting capabilities enable financial planners to develop more effective financial plans and achieve better outcomes for their clients.
Cost Efficiency and Scalability
AI and machine learning contribute to cost efficiency and scalability in financial operations. By automating processes and improving data analysis, financial institutions can reduce operational costs and increase efficiency. These technologies also enable financial institutions to scale their operations without a proportional increase in costs. As a result, financial institutions can expand their services and reach a larger customer base while maintaining profitability.
Implementing AI-Driven Financial Strategies
Understanding the Role of AI in Financial Strategies
AI technologies are transforming the landscape of financial planning and analysis by providing advanced tools for data analysis, forecasting, and decision-making. These technologies enable financial professionals to process vast amounts of data quickly and accurately, uncovering insights that were previously inaccessible. AI can identify patterns and trends in financial data, allowing for more informed strategic decisions.
Key Components of AI-Driven Financial Strategies
Data Collection and Integration
The foundation of any AI-driven financial strategy is robust data collection and integration. Financial institutions must gather data from various sources, including market trends, customer behavior, and internal financial metrics. This data must be integrated into a centralized system where AI algorithms can access and analyze it effectively.
Machine Learning Models
Machine learning models are at the heart of AI-driven financial strategies. These models can be trained to predict financial outcomes, assess risks, and optimize investment portfolios. By continuously learning from new data, machine learning models improve their accuracy over time, providing more reliable insights for financial planning.
Predictive Analytics
Predictive analytics uses AI to forecast future financial trends and outcomes. By analyzing historical data and identifying patterns, predictive analytics can help financial planners anticipate market changes, customer needs, and potential risks. This foresight allows for proactive strategy adjustments and better resource allocation.
Implementing AI in Financial Planning
Identifying Strategic Objectives
Before implementing AI, organizations must clearly define their strategic objectives. This involves understanding what they aim to achieve with AI, such as improving forecasting accuracy, enhancing risk management, or optimizing investment strategies. Clear objectives guide the selection and implementation of AI technologies.
Selecting the Right AI Tools
Choosing the appropriate AI tools is crucial for successful implementation. Organizations should evaluate different AI platforms and solutions based on their specific needs and capabilities. Factors to consider include ease of integration, scalability, and the ability to handle the organization’s data volume and complexity.
Building a Skilled Team
Implementing AI-driven strategies requires a team with expertise in both finance and AI technologies. Financial analysts, data scientists, and IT professionals must collaborate to develop and maintain AI models. Continuous training and development are essential to keep the team updated on the latest AI advancements and financial trends.
Overcoming Challenges in AI Implementation
Data Privacy and Security
One of the primary challenges in implementing AI-driven financial strategies is ensuring data privacy and security. Organizations must comply with regulations and implement robust security measures to protect sensitive financial data. This includes encryption, access controls, and regular security audits.
Managing Change and Adoption
Introducing AI technologies can lead to resistance from employees accustomed to traditional financial planning methods. Effective change management strategies are necessary to encourage adoption and integration of AI tools. This includes providing training, demonstrating the benefits of AI, and fostering a culture of innovation.
Ensuring Model Accuracy and Reliability
AI models must be accurate and reliable to be effective in financial planning. Organizations should implement rigorous testing and validation processes to ensure model performance. Regular updates and recalibration of models are necessary to maintain their accuracy as market conditions and data inputs change.
Challenges and Considerations in Adopting AI Technologies
Data Quality and Management
Ensuring high-quality data is crucial for the successful implementation of AI technologies in financial planning and analysis. Poor data quality can lead to inaccurate predictions and insights, undermining the effectiveness of AI solutions. Organizations must invest in robust data management practices, including data cleansing, validation, and integration, to maintain the integrity and reliability of their data.
Integration with Existing Systems
Integrating AI technologies with existing financial systems can be complex and resource-intensive. Legacy systems may not be compatible with modern AI tools, requiring significant modifications or even complete overhauls. Organizations need to carefully assess their current infrastructure and plan for seamless integration to avoid disruptions and ensure that AI solutions can operate effectively within their existing technological ecosystem.
Talent and Skill Gaps
The adoption of AI technologies necessitates a workforce with specialized skills in data science, machine learning, and AI development. However, there is a notable shortage of professionals with these skills, making it challenging for organizations to find and retain the right talent. Companies must invest in training and development programs to upskill their existing workforce and attract new talent to bridge this gap.
Ethical and Regulatory Considerations
AI technologies in financial planning and analysis must adhere to ethical standards and regulatory requirements. Organizations need to ensure that their AI systems are transparent, fair, and unbiased, avoiding any potential discrimination or ethical breaches. Compliance with data protection regulations, such as GDPR, is also essential to safeguard customer data and maintain trust.
Cost and Resource Allocation
Implementing AI technologies can be costly, requiring significant investment in software, hardware, and human resources. Organizations must carefully evaluate the cost-benefit ratio and allocate resources strategically to ensure that the adoption of AI technologies delivers tangible value. This includes considering the long-term maintenance and scalability of AI solutions.
Change Management and Organizational Culture
The introduction of AI technologies can lead to significant changes in workflows and processes, necessitating effective change management strategies. Organizations must foster a culture of innovation and adaptability, encouraging employees to embrace new technologies and ways of working. Clear communication and stakeholder engagement are critical to overcoming resistance and ensuring a smooth transition.
Security and Privacy Concerns
AI technologies often involve the processing of large volumes of sensitive financial data, raising concerns about data security and privacy. Organizations must implement robust cybersecurity measures to protect against data breaches and unauthorized access. This includes encryption, access controls, and regular security audits to safeguard both organizational and customer data.
Scalability and Flexibility
As organizations grow and their needs evolve, AI solutions must be scalable and flexible to accommodate changing requirements. This involves selecting technologies and platforms that can easily adapt to increased data volumes, new data sources, and evolving analytical needs. Organizations must plan for scalability from the outset to ensure that their AI investments remain relevant and effective over time.
Case Studies: Success Stories in Financial Planning
Leveraging AI for Predictive Analytics in Budgeting
Company A: Transforming Budget Forecasting
Company A, a multinational corporation, implemented AI-driven predictive analytics to enhance its budgeting process. By integrating machine learning algorithms, the company was able to analyze historical financial data and identify patterns that were previously unnoticed. This approach allowed for more accurate budget forecasts, reducing the variance between projected and actual financial outcomes by 20%. The AI system also provided real-time updates, enabling the finance team to make informed decisions swiftly.
Company B: Enhancing Scenario Planning
Company B, a leading financial services firm, utilized AI to improve its scenario planning capabilities. By simulating various economic conditions and their potential impacts on the business, the company could prepare more robust financial plans. The AI models considered a wide range of variables, including market trends and geopolitical events, to provide a comprehensive view of possible future scenarios. This proactive approach helped the company mitigate risks and seize opportunities more effectively.
Machine Learning in Investment Portfolio Optimization
Company C: Revolutionizing Asset Allocation
Company C, an investment management firm, adopted machine learning techniques to optimize its clients’ investment portfolios. By analyzing vast amounts of market data, the machine learning models identified optimal asset allocation strategies tailored to individual risk profiles. This personalized approach resulted in a 15% increase in portfolio returns over a two-year period. The firm also used AI to continuously monitor and adjust portfolios in response to market changes, ensuring sustained performance.
Company D: Enhancing Risk Management
Company D, a hedge fund, implemented machine learning algorithms to enhance its risk management processes. The AI system was designed to detect early warning signs of market volatility and adjust investment strategies accordingly. By leveraging machine learning, the fund reduced its exposure to high-risk assets and improved its overall risk-adjusted returns. This strategic use of AI not only safeguarded the fund’s assets but also instilled greater confidence among investors.
AI-Driven Financial Reporting and Compliance
Company E: Streamlining Financial Reporting
Company E, a global manufacturing company, integrated AI into its financial reporting processes to streamline operations and ensure compliance. The AI system automated data collection and analysis, significantly reducing the time required to generate financial reports. This automation also minimized human errors, enhancing the accuracy and reliability of the reports. As a result, the company achieved a 30% reduction in reporting time and improved its compliance with regulatory standards.
Company F: Enhancing Audit Processes
Company F, a large auditing firm, employed AI to enhance its audit processes. The AI tools were used to analyze large datasets, identify anomalies, and assess compliance with financial regulations. This technology enabled auditors to focus on high-risk areas and provide more insightful recommendations to clients. The use of AI in auditing not only improved the efficiency of the audit process but also increased the firm’s capacity to handle a larger volume of audits without compromising quality.
The Future of AI and Machine Learning in Financial Analysis
Enhanced Predictive Analytics
AI and machine learning are set to revolutionize predictive analytics in financial analysis. By leveraging vast datasets, these technologies can identify patterns and trends that were previously undetectable. This capability allows financial analysts to make more accurate forecasts regarding market trends, investment opportunities, and risk management. Machine learning algorithms can continuously learn from new data, improving their predictive accuracy over time and providing financial institutions with a competitive edge.
Real-time Data Processing
The future of financial analysis will increasingly rely on real-time data processing powered by AI. As financial markets become more dynamic, the ability to process and analyze data in real-time will be crucial. AI systems can quickly process large volumes of data, providing analysts with up-to-the-minute insights. This capability will enable more timely decision-making, allowing financial professionals to respond swiftly to market changes and emerging opportunities.
Automation of Routine Tasks
AI and machine learning will continue to automate routine tasks in financial analysis, freeing up analysts to focus on more strategic activities. Tasks such as data entry, report generation, and basic analysis can be efficiently handled by AI systems. This automation not only increases efficiency but also reduces the risk of human error. As a result, financial analysts can dedicate more time to complex problem-solving and strategic planning.
Improved Risk Management
Risk management is a critical component of financial analysis, and AI is poised to enhance this area significantly. Machine learning algorithms can analyze historical data to identify potential risks and predict future risk scenarios. These insights enable financial institutions to develop more robust risk management strategies. AI can also monitor real-time data to detect anomalies and potential threats, allowing for proactive risk mitigation.
Personalized Financial Advice
AI and machine learning will enable the delivery of personalized financial advice at scale. By analyzing individual financial data and preferences, AI systems can provide tailored recommendations for investments, savings, and financial planning. This personalization enhances customer satisfaction and engagement, as clients receive advice that aligns with their unique financial goals and circumstances.
Integration with Blockchain Technology
The integration of AI and machine learning with blockchain technology holds significant potential for the future of financial analysis. Blockchain provides a secure and transparent platform for financial transactions, and AI can enhance its capabilities by analyzing blockchain data for insights. This integration can improve transaction verification, fraud detection, and compliance with regulatory requirements, leading to more secure and efficient financial systems.
Ethical and Regulatory Considerations
As AI and machine learning become more prevalent in financial analysis, ethical and regulatory considerations will play a crucial role. Ensuring the ethical use of AI involves addressing issues such as data privacy, algorithmic bias, and transparency. Regulatory bodies will need to establish guidelines and frameworks to govern the use of AI in financial services, ensuring that these technologies are used responsibly and do not compromise consumer trust.
Conclusion: Embracing the New Frontier in Finance
The Transformative Power of AI and Machine Learning
The integration of AI and machine learning into financial planning and analysis represents a transformative shift in how financial professionals approach their work. These technologies offer unprecedented capabilities in data processing, predictive analytics, and decision-making. By leveraging AI, financial planners can analyze vast datasets with greater speed and accuracy, uncovering insights that were previously inaccessible. Machine learning algorithms can identify patterns and trends that inform strategic decisions, enabling organizations to anticipate market changes and adjust their strategies proactively.
Overcoming Challenges and Building Trust
While the benefits of AI and machine learning are clear, the transition to these technologies is not without challenges. Financial institutions must address concerns related to data privacy, security, and ethical considerations. Building trust in AI systems is crucial, as stakeholders need assurance that these technologies are reliable and unbiased. Financial professionals must work closely with data scientists and IT teams to ensure that AI systems are transparent and that their outputs are explainable. This collaboration is essential to overcoming skepticism and fostering a culture of trust and innovation.
The Role of Human Expertise
Despite the advanced capabilities of AI and machine learning, human expertise remains indispensable in financial planning and analysis. Financial professionals bring critical thinking, contextual understanding, and ethical judgment that machines cannot replicate. The role of humans is evolving from performing routine tasks to focusing on strategic decision-making and relationship management. By embracing AI as a tool that enhances their capabilities, financial professionals can focus on higher-value activities, such as interpreting complex data insights and advising clients on strategic financial decisions.
Preparing for the Future
To fully embrace the new frontier in finance, organizations must invest in the necessary infrastructure, talent, and training. This includes upgrading technology systems, hiring data scientists, and providing ongoing education for financial professionals to develop AI literacy. Organizations should foster a culture of continuous learning and innovation, encouraging employees to experiment with new technologies and approaches. By preparing for the future, financial institutions can position themselves at the forefront of industry advancements, driving growth and competitive advantage.
A Call to Action for Financial Leaders
Financial leaders play a pivotal role in guiding their organizations through this transformative period. They must champion the adoption of AI and machine learning, setting a vision for how these technologies will be integrated into financial planning and analysis. Leaders should prioritize strategic investments in technology and talent, while also fostering a culture of collaboration and innovation. By taking proactive steps to embrace AI and machine learning, financial leaders can ensure their organizations are well-equipped to navigate the complexities of the modern financial landscape.
Related posts:
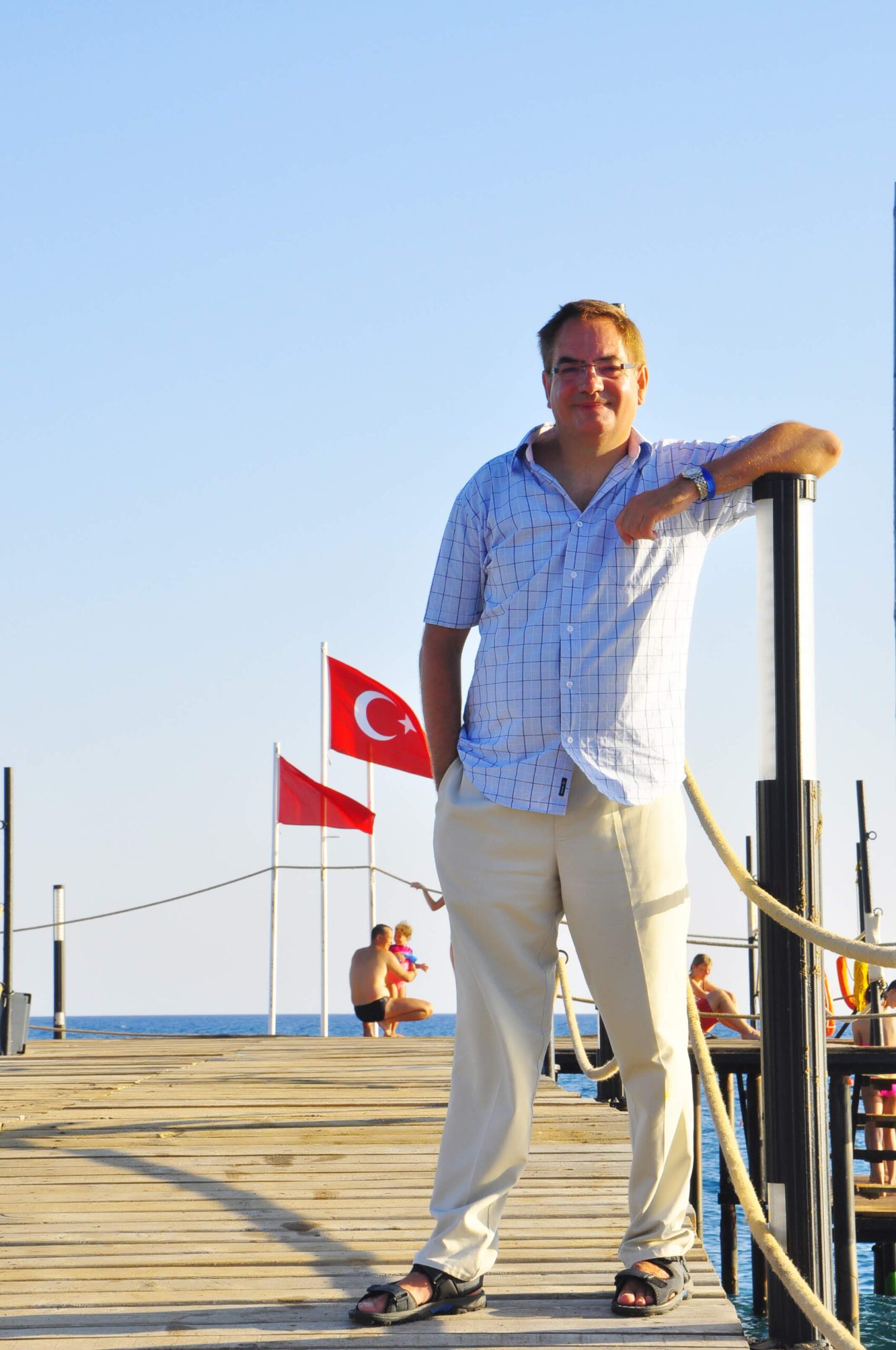
Adrian Lawrence FCA with over 25 years of experience as a finance leader and a Chartered Accountant, BSc graduate from Queen Mary College, University of London.
I help my clients achieve their growth and success goals by delivering value and results in areas such as Financial Modelling, Finance Raising, M&A, Due Diligence, cash flow management, and reporting. I am passionate about supporting SMEs and entrepreneurs with reliable and professional Chief Financial Officer or Finance Director services.